Research Results
Kyowoon Lee, Artyom Stitsyuk, Gunu Jho, Inchul Hwang and Jaesik Choi
Recent advances in Text-to-Speech (TTS) have significantly improved speech naturalness, increasing the demand for precise prosody control and mispronunciation correction. Existing approaches for prosody manipulation often depend on specialized modules or additional training, limiting their capacity for post-hoc adjustments. Similarly, traditional mispronunciation correction relies on grapheme-to-phoneme dictionaries, making it less practical in low-resource settings. We introduce Counterfactual Activation Editing, a model-agnostic method that manipulates internal representations in a pre-trained TTS model to achieve post-hoc control of prosody and pronunciation. Experimental results show that our method effectively adjusts prosodic features and corrects mispronunciations while preserving synthesis quality. This opens the door to inference-time refinement of TTS outputs without retraining, bridging the gap between pre-trained TTS models and editable speech synthesis.
Kyowoon Lee and Jaesik Choi
Recent advances in diffusion-based generative modeling have demonstrated significant promise in tackling long-horizon, sparse-reward tasks by leveraging offline datasets. While these approaches have achieved promising results, their reliability remains inconsistent due to the inherent stochastic risk of producing infeasible trajectories, limiting their applicability in safety-critical applications. We identify that the primary cause of these failures is inaccurate guidance during the sampling procedure, and demonstrate the existence of manifold deviation by deriving a lower bound on the guidance gap. To address this challenge, we propose Local Manifold Approximation and Projection (LoMAP), a training-free method that projects the guided sample onto a low-rank subspace approximated from offline datasets, preventing infeasible trajectory generation. We validate our approach on standard offline reinforcement learning benchmarks that involve challenging long-horizon planning. Furthermore, we show that, as a standalone module, LoMAP can be incorporated into the hierarchical diffusion planner, providing further performance enhancements.
Jiyeon Han*, Dahee Kwon*, Gayoung Lee, Junho Kim and Jaesik Choi
Recent text-to-image generative models, particularly Stable Diffusion and its distilled variants, have achieved impressive fidelity and strong text-image alignment. However, their creative capability remains constrained, as including `creative’ in prompts seldom yields the desired results. In this paper, we introduce C3 (Creative Concept Catalyst), a training-free approach designed to enhance creativity in Stable Diffusion-based models. C3 selectively amplifies features during the denoising process to foster more creative outputs. We offer practical guidelines for choosing amplification factors based on two main aspects of creativity. C3 is the first study to enhance creativity in diffusion models without extensive computational costs. We demonstrate its effectiveness across various Stable Diffusion-based models.
Seongyeop Jeong, June Sig Sung, Inchul Hwang and Jaesik Choi
Recent advances in deep learning technology have enabled high-quality speech synthesis, and text-to-speech models are widely used in a variety of applications. However, even stateof-the-art models still produce artificial speech, highlighting the need to correct errors in synthesized speech. Traditional speech correction methodologies face a number of challenges, such as the inefficiency of manually specifying errors, the need to retrain models, and the need for additional data to correct synthesized speech errors. In this paper, we present a novel approach that detects and corrects contextual errors within a model to improve synthetic speech without requiring additional resources or model retraining. Specifically, we analyze the inherent limitations of neural network encoders responsible for contextualizing input sentences and propose a method that automatically identifies abnormal encoder context vectors. We also introduce a correction algorithm that enhances the quality of speech by correcting the incorrect relationships between phonemes that cause abnormal encoder context vectors. Our algorithm demonstrated a 25.86% and 4.69% reduction in alignment errors and a 2.25% and 0.63% improvement in objective metrics such as Frechet Wav2Vec ´ distance for the Tacotron2 and VITS models. In addition, our algorithm improved the comparative mean opinion scores, a subjective evaluation, by 1.34 and 0.52. The results support the feasibility of a novel approach to identify, correct, and improve defects automatically in neural speech synthesis models. The results support the feasibility of a novel approach to identify, correct, and improve defects automatically in neural speech synthesis models.
Wonjoon Chang, Myeongjin Lee, Jaesik Choi
We study causal interactions for payoff allocation in cooperative game theory, including quantifying feature attribution for deep learning models. Most feature attribution methods mainly stem from the criteria of the Shapley value, which assigns fair payoffs to players based on their expected contribution in a cooperative game. However, interactions between players in the game do not explicitly appear in the original formulation of the Shapley value. In this work, we reformulate the Shapley value to clarify the role of interactions and discuss implicit assumptions from a game-theoretical perspective. Our theoretical analysis demonstrates that when negative interactions exist—common in deep learning models—the efficiency axiom can lead to the undervaluation of attributions or payoffs. We suggest a new allocation rule that decomposes contributions into interactions and aggregates positive parts for non-convex games. Furthermore, we propose an approximation algorithm to reduce the cost of interaction computation which can be applied to differentiable functions such as deep learning models. Our approach mitigates counterintuitive attribution outcomes observed in existing methods, ensuring that features critical to a model’s decision receive appropriate attribution.
Anh Tong, Thanh Nguyen-Tang, Dongeun Lee, Duc Nguyen, Toan Tran, David Leo Wright Hall, Cheongwoong Kang and Jaesik Choi
Recent advancements in large language models (LLMs) based on transformer architectures have sparked significant interest in understanding their inner workings. In this paper, we introduce a novel approach to modeling transformer architectures using highly flexible non-autonomous neural ordinary differential equations (ODEs). Our proposed model parameterizes all weights of attention and feedforward blocks through neural networks, expressing these weights as functions of a continuous layer index. Through spectral analysis of the model’s dynamics, we uncover an increase in eigenvalue magnitude that challenges the weightsharing assumption prevalent in existing theoretical studies. We also leverage the Lyapunov exponent to examine token-level sensitivity, enhancing model interpretability. Our neural ODE transformer demonstrates performance comparable to or better than vanilla transformers across various configurations and datasets, while offering flexible fine-tuning capabilities that can adapt to different architectural constraints.
Artyom Stitsyuk and Jaesik Choi
In this work, we design eXponential Patch (xPatch for short), a novel dual-stream architecture that utilizes exponential decomposition. Inspired by the classical exponential smoothing approaches, xPatch introduces the innovative seasonal-trend exponential decomposition module. Additionally, we propose a dual-flow architecture that consists of an MLP-based linear stream and a CNN-based non-linear stream. This model investigates the benefits of employing patching and channel-independence techniques within a non-transformer model. Finally, we develop a robust arctangent loss function and a sigmoid learning rate adjustment scheme, which prevent overfitting and boost forecasting performance.
Subeen Lee, Jiyeon Han, Soyeon Kim and Jaesik Choi
This study proposes a novel approach for generating diverse rare samples from high-resolution image datasets with pretrained GANs. Our method employs gradient-based optimization of latent vectors within a multi-objective framework and utilizes normalizing flows for density estimation on the feature space. This enables the generation of diverse rare images, with controllable parameters for rarity, diversity, and similarity to a reference image. We demonstrate the effectiveness of our approach both qualitatively and quantitatively across various datasets and GANs without retraining or fine-tuning the pretrained GANs.
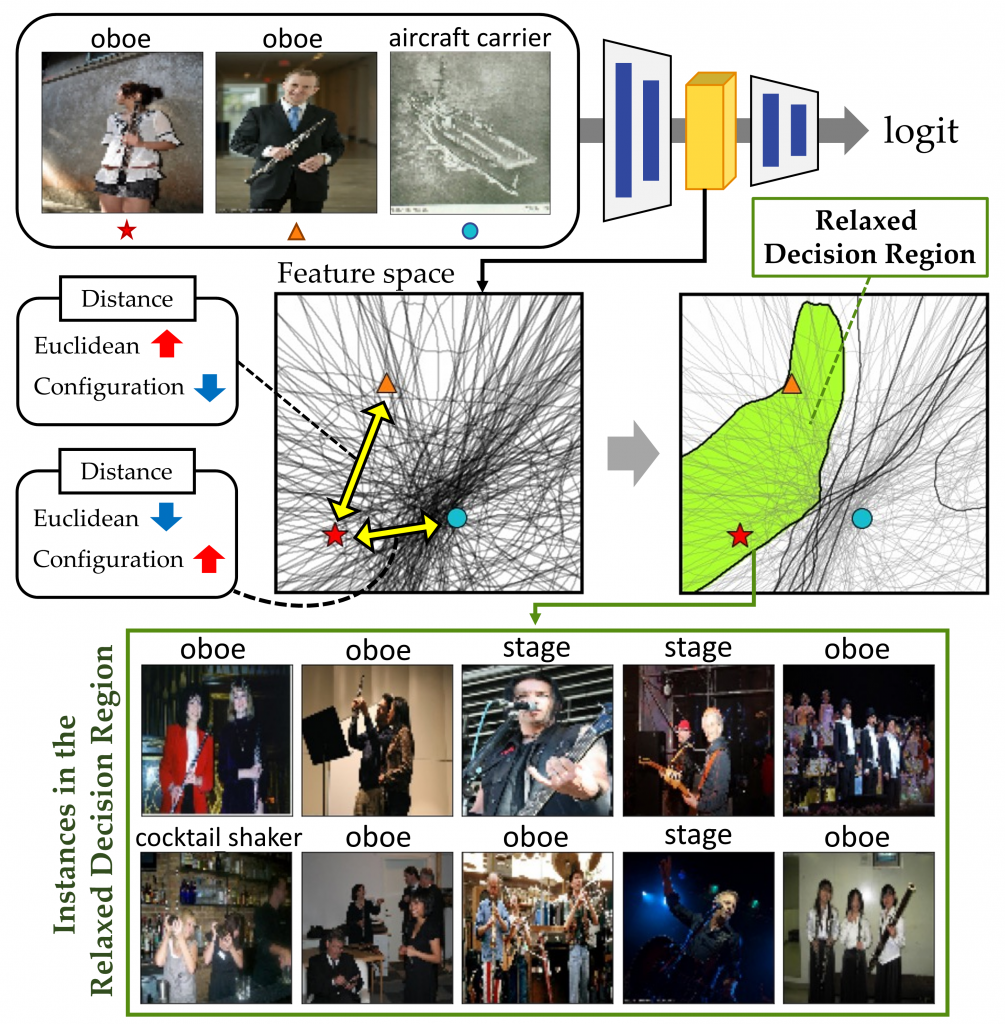
Wonjoon Chang*, Dahee Kwon* and Jaesik Choi
Understanding intermediate representations of the concepts learned by deep learning classifiers is indispensable for interpreting general model behaviors. In this paper, we propose a novel unsupervised method for discovering distributed representations of concepts by selecting a principal subset of neurons. Our empirical findings demonstrate that instances with similar neuron activation states tend to share coherent concepts. Based on the observations, the proposed method selects principal neurons that construct an interpretable region, namely a Relaxed Decision Region (RDR), encompassing instances with coherent concepts in the feature space.
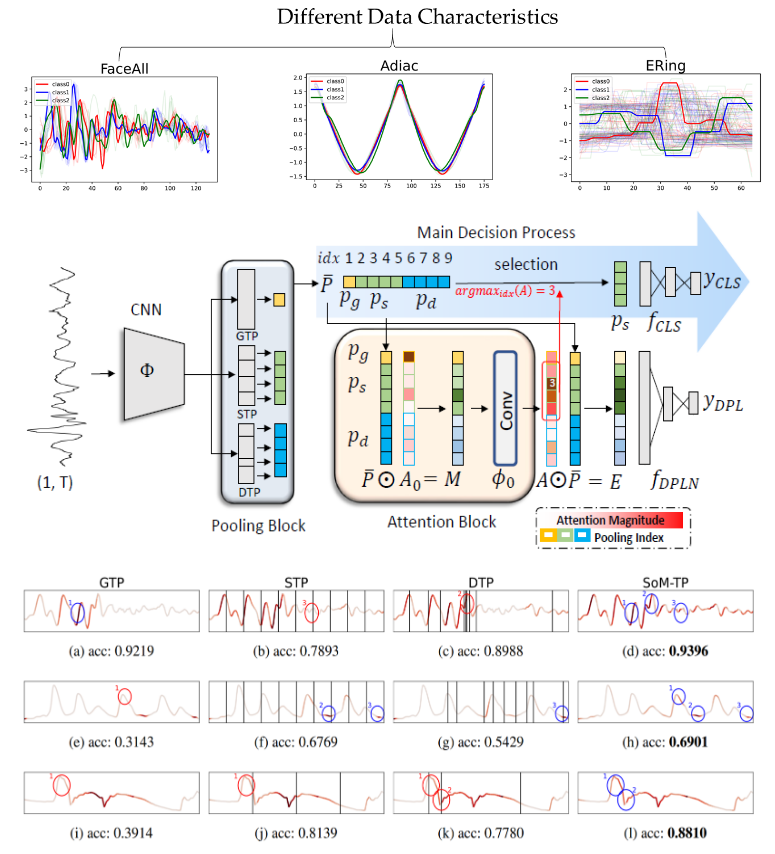
Jihyeon Seong, Jungmin Kim and Jeasik Choi
We are finding a temporal pooling automatically reflecting the diverse characteristics of time series data in time series classification task. Previous temporal poolings exhibit data dependency based on segmentation type, and each excels with different time series data characteristics. Selection over Multiple Temporal Poolings (SoM-TP) employs selection ensemble learning to utilize the perspective of each temporal pooling in a data driven way. The diverse perspective learning of SoM-TP addresses the drawbacks of a single pooling perspective while also incorporating the benefits of the best-performing temporal pooling.
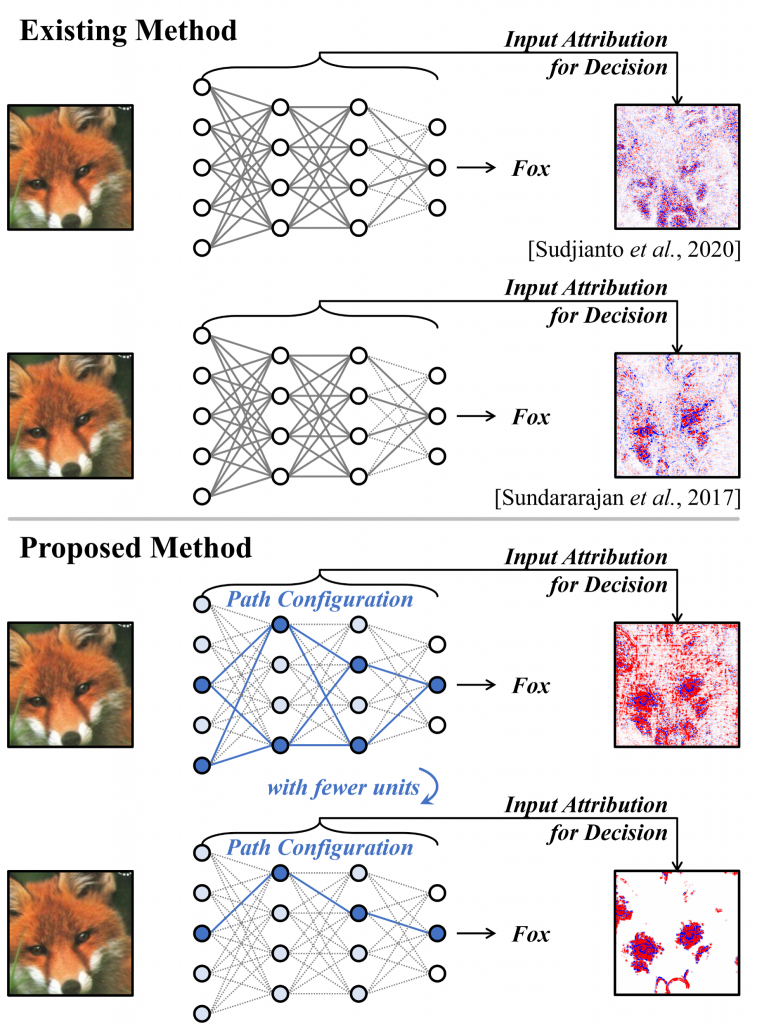
Seongwoo Lim, Won Jo, Joohyung Lee and Jaesik Choi
We decompose the ReLU neural network into components, called paths, based on their locally linear attributes. We identify a piecewise linear model corresponding to each path, and use it to generate input attributions that explain the model’s decisions. Configuration of the paths allow explanations to be generated by concentrating on the important parts, and decomposed across different paths to explain each object and feature.
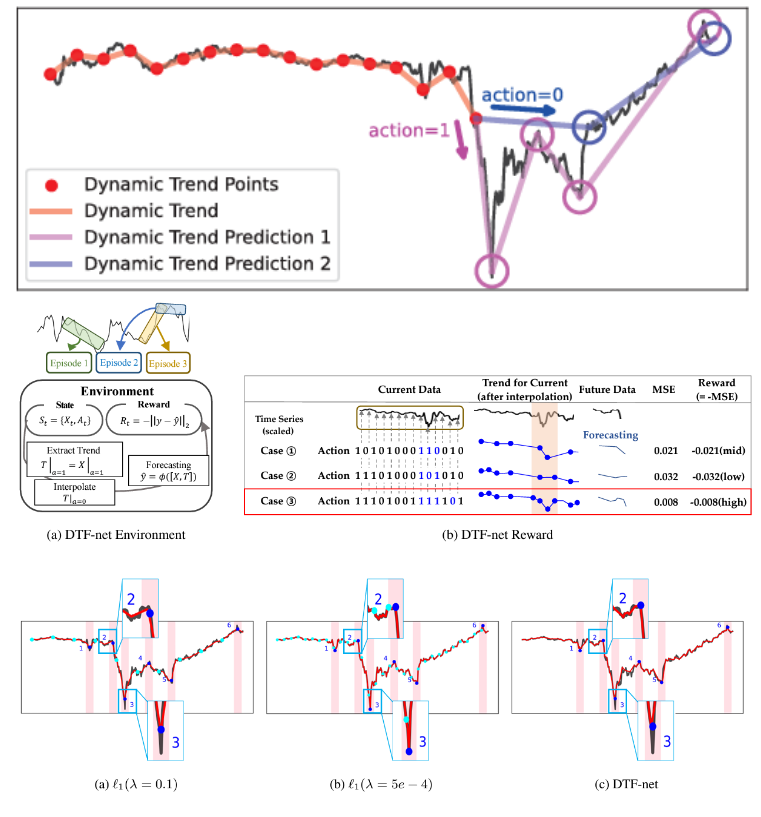
Jihyeon Seong, Sekwang Oh and Jeasik Choi
We are finding important points automatically reflecting extreme values of time series data to improve time series forecasting performance. Previous trend filtering methods ignore extreme values as outliers, which have notable information. Dynamic Trend Filtering network (DTF-net) utilizes RL to capture important points that should be reflected in the trend, including extreme values. With the trend from DTF-net, time series forecasting performance is improved.
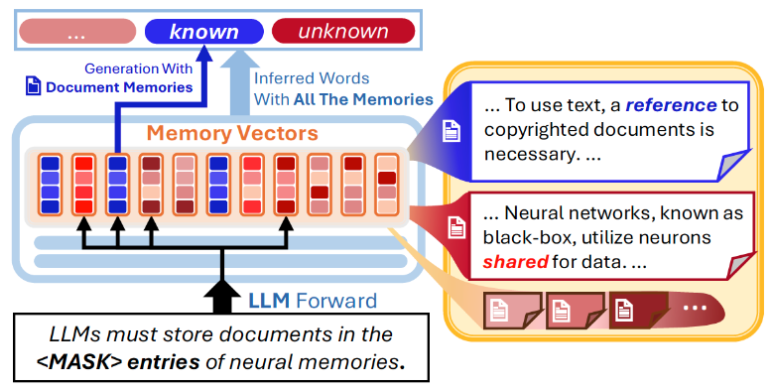
Bumjin Park and Jaesik Choi
In AI, data plays a pivotal role in training. Large language models (LLMs) are trained with massive amounts of documents, and their parameters hold document contents. Recently, several studies identified content-specific locations in LLMs by examining the parameters. Instead of the post hoc interpretation, we propose another approach, document-wise memories, which makes document-wise locations for neural memories in training. The proposed architecture maps document representation to memory entries and filters memory selections in the forward process of LLMs.
of Large Language Models
Cheongwoong Kang and Jaesik Choi
We hypothesize that relying heavily on simple co-occurrence statistics of the pre-training corpora is one of the main factors that cause factual errors. Our results reveal that LLMs are vulnerable to the co-occurrence bias, defined as preferring frequently co-occurred words over the correct answer. Consequently, LLMs struggle to recall facts whose subject and object rarely co-occur in the pre-training dataset although they are seen during finetuning.
Kyowoon Lee*, Seongun Kim* and Jaesik Choi
We propose a method to refine unreliable plans generated by diffusion-based planners for long-horizon tasks. We introduce a “restoration gap” metric to guide and improve these plans and uses an attribution map regularizer to prevent adversarial guidance. The approach is validated on benchmarks and enhances explainability by highlighting error-prone transitions in the generated plans.
Soyeon Kim, Junho Choi, Yeji Choi, Subeen Lee, Artyom Stitsyuk, Minkyoung Park, Seongyeop Jeong, Youhyun baek and Jaesik Choi
As machine learning (ML) becomes more prevalent in meteorological decision-making, user-centered explainable artificial intelligence (XAI) studies have yet to extend into this domain. This study identifies three key requirements for explaining black-box models in meteorology: model bias in different rainfall scenarios, model reasoning, and output confidence. Appropriate XAI methods are mapped to these requirements and evaluated both quantitatively and qualitatively. The results show that intuitive explanations improve decision-making utility and user trust, highlighting the need for user-centered XAI to enhance the usability of AI systems in practice.
for Reliable Input Attribution via Randomized Path Sampling
Giyoung Jeon, Haedong Jeong and Jaesik Choi
In this paper, we tackle this issue by estimating the distribution of the possible attributions according to the integrating path selection. We show that such noisy attribution can be reduced by aggregating attributions from the multiple paths instead of using a single path. Using multiple input attributions obtained from randomized path, we propose a novel attribution measure using the distribution of attributions at each input features.
Seongun Kim, Kyowoon Lee and Jaesik Choi
We introduce Variational Curriculum RL (VCRL), a method for unsupervised skill discovery in reinforcement learning by using intrinsic rewards and curriculum learning. The proposed Value Uncertainty Variational Curriculum (VUVC) accelerates skill acquisition and improves state coverage compared to uniform approaches. The approach is validated on complex tasks, showing improved sample efficiency and successful real-world robotic navigation in a zero-shot setup.
Jiyeon Han, Hwanil Choi, Yunjey Choi, Junho Kim, Jung-Woo Ha and Jaesik Choi
In this work, we propose a new evaluation metric, called ‘rarity score’, to measure the individual rarity of each image synthesized by generative models. We first show empirical observation that common samples are close to each other and rare samples are far from each other in nearest-neighbor distances of feature space. We then use our metric to demonstrate that the extent to which different generative models produce rare images can be effectively compared. We also propose a method to compare rarities between datasets that share the same concept such as CelebA-HQ and FFHQ.
Kyowoon Lee*, Seongun Kim* and Jaesik Choi
We propose a hierarchical framework for robotic navigation that learns a family of diverse low-level policies and a high-level policy to deploy them adaptively. Instead of relying on a fixed reward function, the method learns multiple navigation skills with varied reward functions and selects the most suitable one in real time. The approach is validated in simulation and real-world settings, demonstrating both adaptability and explainability of the agent’s behavior.
Giyoung Jeon*, Haedong Jeong* and Jaesik Choi
In this paper, we present an explorative sampling algorithm to analyze generation mechanism of DGNNs. Our method efficiently obtains samples with identical attributes from a query image in a perspective of the trained model. We define generative boundaries which determine the activation of nodes in the internal layer and probe inside the model with this information.