Research Projects
XAI Center
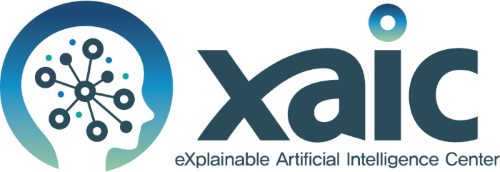
The XAI center is developing new or modified machine learning techniques to produce explainable AI models. The explainable AI models provide effective explanation for human to understand the reasons of decision making via machine learning and statistical inference based on real-world data. The center highly contributes to Medical and Financial industry, which obtains high advantage by adopting AI but high risk follows without explainability.
Unmanned Swarm Cyber Physical System
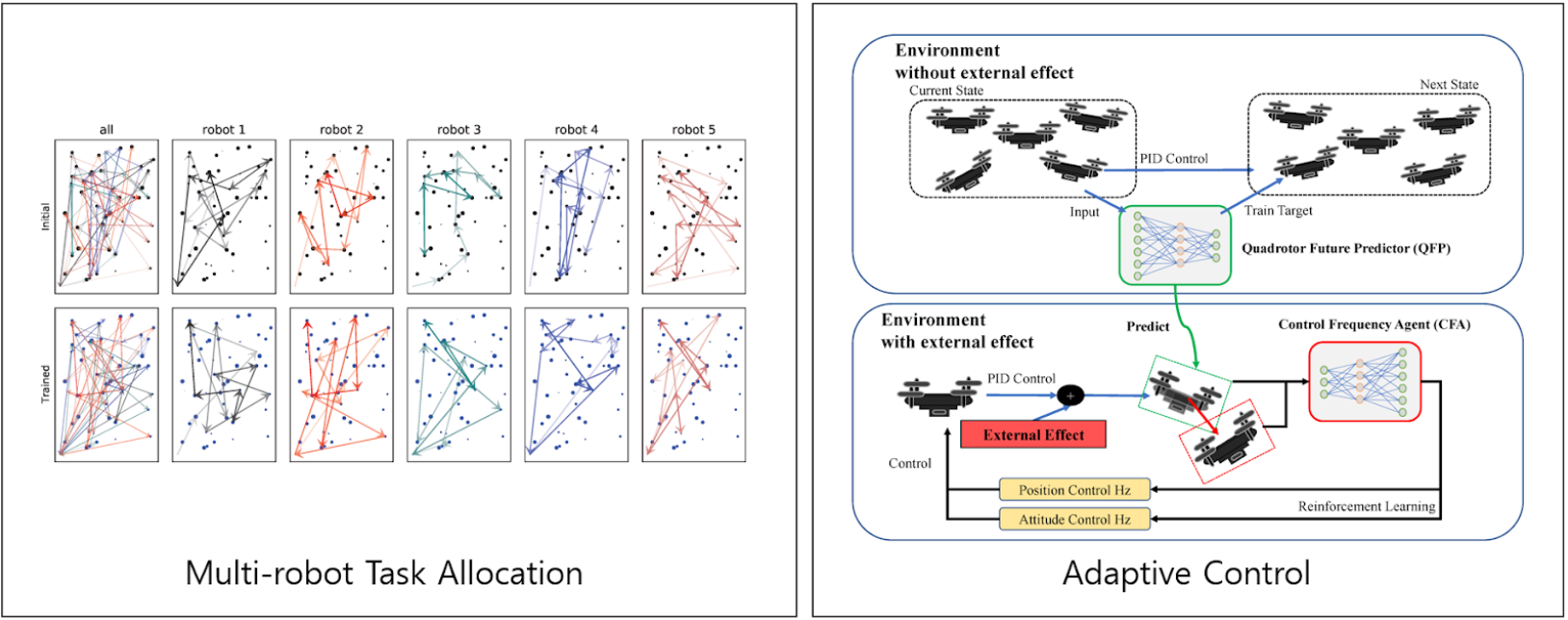
In this project, we develop reinforcement learning algorithms tailored for the seamless navigation and operation of drones and mobile robots. The main goals include crafting adaptive control strategies that stand resilient against the unpredictable shifts in dynamics, such as variations in payload and the challenges posed by changing wind conditions. A significant pillar of this project is the development of advanced multi-agent systems, designed to facilitate seamless cooperation among robots, enabling them to tackle complex tasks in unison. Through this project, we aim to push the boundaries of robotic capabilities, enhancing their autonomy and efficiency in dynamic environments.
Explainable AI for Weather Forecast AI Models

In this project, we are focused on designing an explainable AI algorithm, grounded in user experiments, alongside an intuitive human-computer interaction (HCI) interface tailored for forecasters. We aim to demystify AI decision-making processes in weather prediction, enhancing trust and usability for professionals in the field.
Explainable AKI Prediction and Prevention System

In this project, we develop an AI-driven system aimed at forecasting Acute Kidney Injury (AKI) in hospitalized patients. This system not only predicts potential AKI incidents but also employs eXplainable AI (XAI) techniques to illuminate modifiable risk factors for attending physicians. By providing actionable insights, the system empowers healthcare professionals to implement preventative measures, thereby aiming to lower the incidence rate of AKI through timely intervention.
ADD-XAI
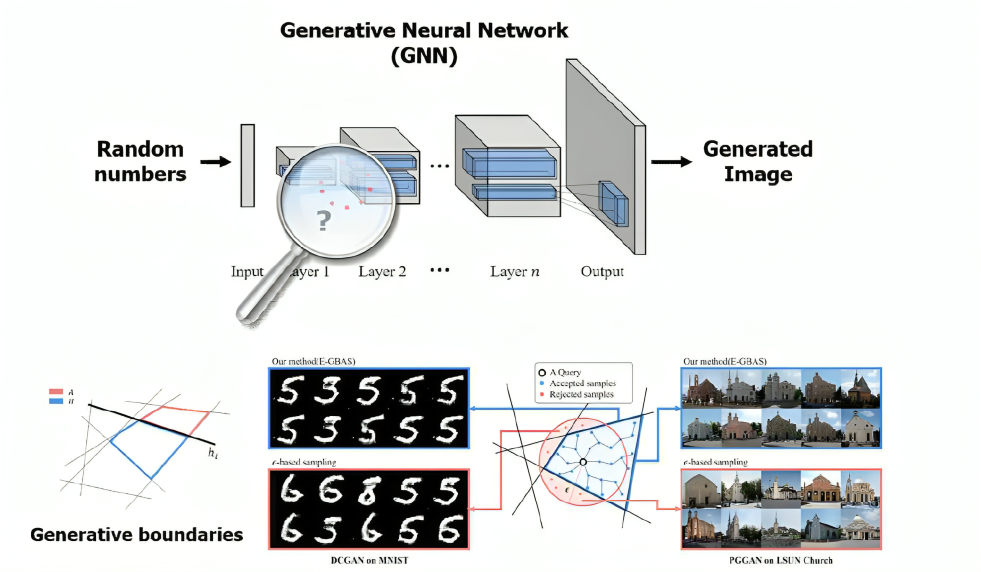
This project aims to automatically detect and uncover bugs in deep learning models, enhancing their robustness and reliability. In the initial phase, we focused on debugging the internals of adversarial generative deep neural networks. We achieved this by extracting samples with similar characteristics and then repairing and detecting mis-trained nodes in an unsupervised manner. The scope of this research has since expanded to encompass general deep neural networks.
KAIST-NAVER Hypercreative AI
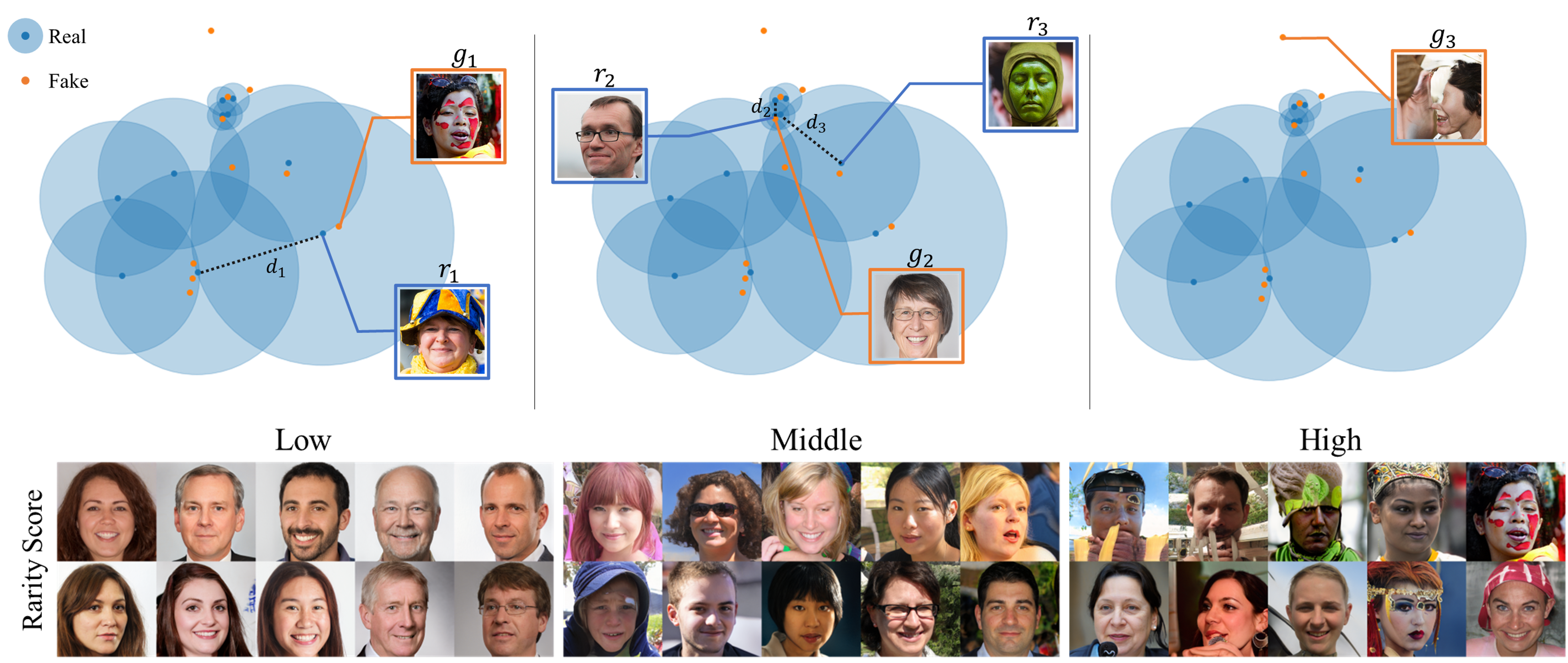
The Hypercreative AI project endeavors to develop AI models that facilitate the generation of creative outcomes. Within this project, our research team is dedicated to discerning levels of creativity, implementing effective filters for creative generations, and enhancing the generation of creative outputs. Additionally, we are keen to explore methods for training a model that exhibits robustness in generating rare samples.
Projected are supported by
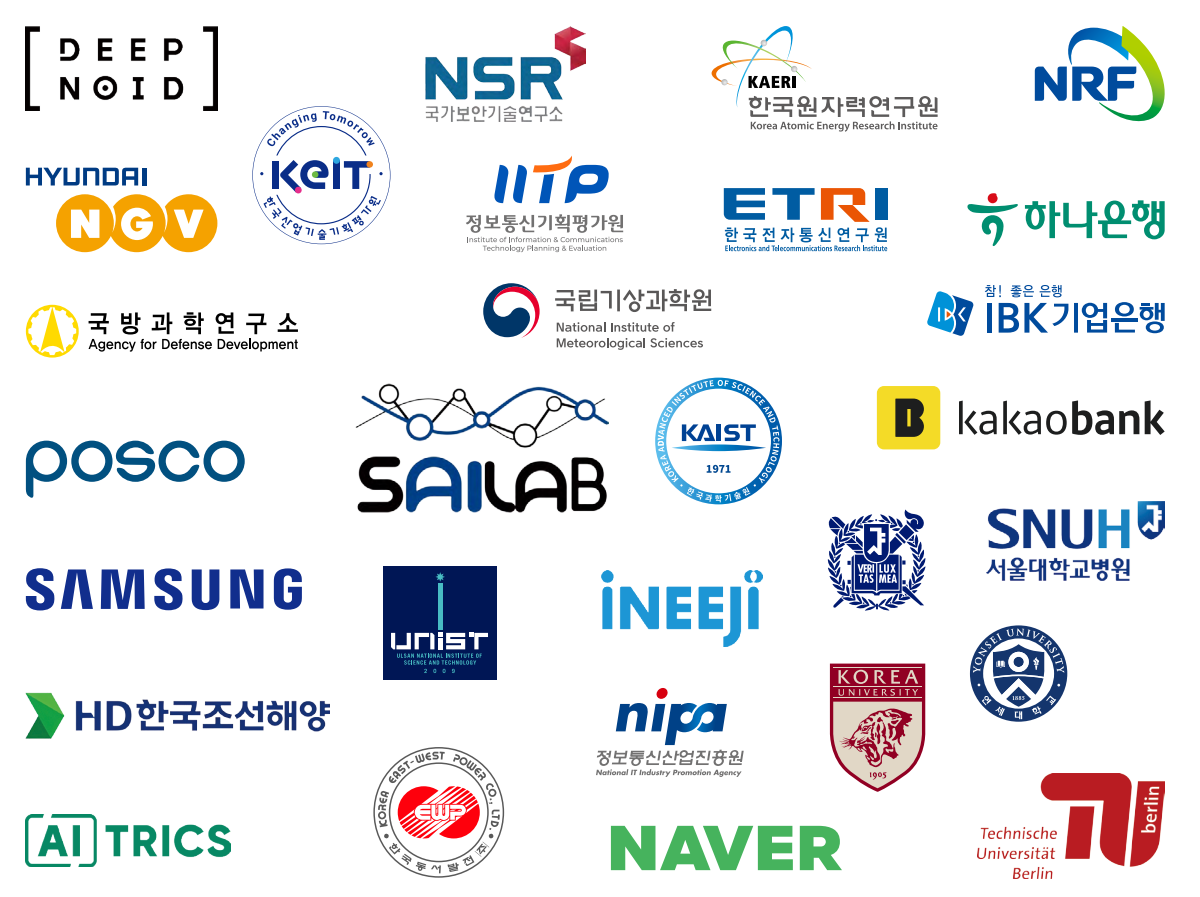